Product recommendation engines have elevated the online browsing and purchasing experience. The most common example of what product recommendation engines do is the “you might also like” section you're shown as you're browsing on an e-commerce website.
Or when you see ads on your social media feed from the brands for similar or complementary products to the ones you've just ordered.
Behind this lie product recommendation engines that run rules-based algorithms to serve up the most relevant offering to consumers.
Personalization Strategies with Product Recommendations
Product recommendation engines analyze data about visitors to show them other relevant content, products, or services that likely would interest them. However, serving up relevant items to individual users to drive conversion rates is an involved process.
Businesses looking to implement a product recommendation engine should use various data points such as purchase history, session duration, on-site behavior, preferences, and feedback to make relevant product recommendations. Therefore, a product recommendation engine cannot generate accurate recommendations without good user data.
What is a Product Recommendation Engine?
A product recommendation engine analyzes user data to make relevant suggestions.
How Do Product Recommendation Engines Work?
Product recommendation engines use complex algorithms, to scan massive amounts of data, including purchase history, browsing behavior, and wish lists to make recommendations.
The recommendation algorithm automatically generates appropriate recommendations based on user information. The software solution then shows the most relevant suggestions for each user.
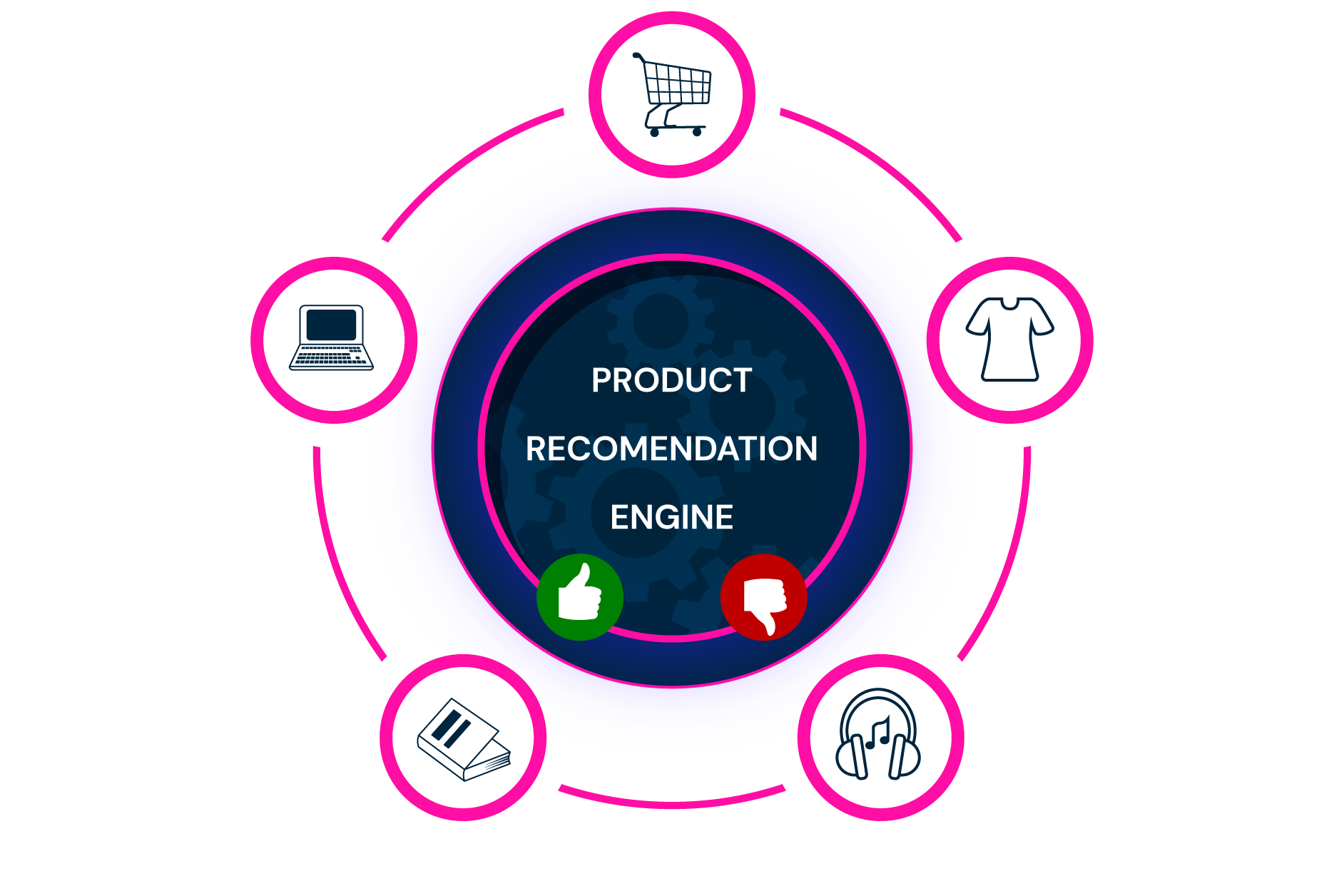
A product recommendation engine is an incredibly powerful software solution that uses sophisticated algorithms to make personalized product recommendations — such as special deals, promotional content, and discounts —to each website visitor.
Product Recommendations Metrics
Companies use various metrics to make product recommendations. These are some of the most common types of customer data businesses use to make product recommendations:
- Browsing & Purchasing Behavior
- Customer ratings
- Most-Viewed Items
- Recently Viewed Products
- Purchase History
- Time-on-page
- Search History
- Wish List
Based on that data, the engine can offer relevant products in which the shopper might be interested. Properly configured recommendation engines accurately anticipate visitor intent and display suggested items in social media ads, marketing emails, or site searches.
Filtering System
Think of a product recommendation engine as a complex filtering system that predicts and displays the products and services a user might want to buy.
Major ecommerce businesses like Amazon use their recommendation engines to increase sales while helping customers find other relevant products without hassle.
Different Product Recommendation Methods
Product recommendation engines vary based on the type of data they use to make product suggestions to customers. Most product recommendation engines fall into these categories:
- Collaborative filtering systems
- Content-based filtering systems
- Hybrid recommendation systems
Let's now take a closer look at how each of these recommendation systems works.
Collaborative Recommendation
The collaborative recommendation engine makes decisions using the preferences of visitors. It typically tracks the purchase and browsing information for a few items by multiple users.
The idea behind this filtering system is that if user A has the same opinion as user B on a topic, then user A is likely to have the same opinion on another topic.
You've probably seen websites that recommend something using the phrase, "people who bought that also bought this."
For example, a visitor browsing running shoes on a sports e-commerce website may see recommendations for items other customers who bought running shoes also bought such as socks or breathable t-shirts for running.
Content-Based Filtering Systems
A content-based recommendation system filters users' preferences and purchasing behavior to suggest products. The algorithms recommend products that are similar to the ones that a user has liked in the past.
This type of filtering system produces the ‘since you bought this product, you’ll also like this product’ recommendations.
Examples
Brands that use a content-based filtering method typically rely on cookies to track users over several visits. This allows them to make recommendations based on user preferences (likes and dislikes).
In this filtering system, rules-based algorithms recommend items that resemble those which the person liked in the past.
YouTube likes to use content-based recommendations. Youtube recommends videos based on the type of content users have previously watched.
Hybrid Recommendation Systems
The hybrid approach combines collaborative and content-based filtering systems. It relies on data sets from groups of similar users along with the past preferences of each user.
Many leading ecommerce businesses use collaborative and content-based recommendations systems.
For instance, an ecommerce business might use customer data on customers who have bought gaming keyboards with an individual buyer who purchased a gaming keyboard.
The recommendation engine can be configured to serve up gaming headsets because other customers who purchased keyboards for gaming also purchased gaming headsets and the individual customer also searched for headsets in the past.
Popular Recommendation Strategies
Here are some of the most popular ways businesses across industries are putting product recommendation engines to work.
Ratings-Based Recommendations
Product reviews play an important role in influencing customers' purchasing decisions.
Customer ratings, product reviews, and feedback are metrics that can be tracked and used to make suggestions. Typically, a product recommendation tool that puts forward suggestions based on user ratings will also consider things like the number of clicks, the length of time a customer spent reading a blog post or looking at a product listing.
Personalized Recommendations
Based on the browsing and purchase history, product recommendation engines can make personalized offers for customers.
Frequently Bought Together Bundle
A popular sales tactic used by online retailers is to display closely related products on the checkout page. This kind of recommendation boosts the shopping experience because it gives customers useful suggestions that complement their purchases.
However, this type of recommendation can also be used in post-purchase (or confirmation emails) to offer products or services that go well with the purchased items.
This recommendation tactic uses consumer behavior in online retail to segment customers into groups with similar tastes and spending habits.
Benefits of Production Recommendation Engines
A product recommendation engine increases brand awareness, sales, customer satisfaction, ROI of marketing strategies. Let's take a closer look at the many benefits of using a product recommendation tool.
Better Click-Through Rates (CTR)
It should come as no surprise that the click-through rate of personalized suggestions is much higher than the click-through rate of generic recommendations.
Average Order Value
Recommendation engines provide great opportunities to cross-sell, upsell, and promote slow-moving inventory.
Properly configured recommendation engines give your site visitors a higher volume of products that are likely to be of use to them.
Bundle Up Products
Putting together several products into one bundle is a proven way of upselling or cross-selling items across different price points and description categories.
Customer behavior and purchase history are very useful metrics that allow a product recommendation tool to recommend relevant items.
Complete the Look Recommendation
The complete the look recommendation tactic is popular with online clothing stores. For instance, as a shopper views a sweater, the recommendation engine offers complimentary items, such as pants and shoes.
When a shopper sees the item within a larger context, the likelihood that they make a purchase increases.
Increase Average Order Value
Whether you want to promote an entire outfit or build the ultimate gaming set-up, ‘complete the look’ bundle recommendations are a proven way of turning smaller transactions into significantly larger ones.
Increasing Customer Retention Rates
Offering product recommendations requires can be difficult. Rules engines can make recommendations based on customers' location or size measurements so as to avoid displaying products that don't come in their size or don't ship to their location.
Generate More Revenue
Obviously, higher conversion rates and more money spent per transaction generate more revenue for the company.
Personalization Makes Great Customer Experiences
Recommendations engines provide customers with highly relevant content based on their, interests, preferences, and purchasing habits. This makes visitors feel known, which improves the customer experience.
According to a recent report, up to 31% of ecommerce site revenue comes from personalized product recommendations.
When consumers are able to find what they’re looking for along with other relevant items, it enhances their customer experience.
Drive Business Growth with Higson
Product recommendation engines provide buyers personalized recommendations at every step of their customer journey.
They enable shoppers to view relevant products using their personal preferences and current session intent, thereby improving retention rates and conversions. With Higson, businesses can build their own recommendation strategy optimized for their specific business goals.
Higson optimizes product recommendations by executing rules that take into account any number of data points, such as purchase history and geo-targeting parameters, to provide a seamless customer journey. For example, the recommendation filters can ensure only items available in the customer's size will be displayed.
Bottom Line
A product recommendation engine is designed to offer a better overall customer experience using customized suggestions for items that they otherwise might not have come across by themselves.
We’ve configured Higson BREto help optimize the customer experience and boost conversion rates. If you’re looking for proven ways to improve the shopping experience for your customers, schedule your free call with us today.
Our user-friendly product recommendation engine is designed to give subject-matter experts the power to make changes to the recommendation algorithm on the fly. No technical skills are needed — whether you're a senior digital product manager, sales rep, or CTO — Higson enables companies to leverage the expertise of their team members.
Whether you only need straightforward recommendations to help out with slow-moving items or you run a major online retail business, Higson has all the necessary features to help you realize your business goals.